Data-Driven Insights in Crypto Trading: Navigating the Volatile Cryptocurrency Landscape
The digital revolution has ushered in an era of cryptocurrencies, a novel form of decentralized digital assets. With their rise, the financial world has witnessed unprecedented volatility, making the crypto market both an opportunity and a challenge for traders. In this landscape, data-driven insights have emerged as a beacon, guiding traders through the tumultuous waves of crypto trading. This article delves deep into the transformative role of data-driven insights in navigating the volatile cryptocurrency landscape.
The Rise of Cryptocurrencies
Cryptocurrencies, led by Bitcoin, have disrupted traditional financial systems. Their decentralized nature, free from central authority interference, has made them both appealing and unpredictable. As of today, there are over 10,000 different cryptocurrencies, each with its unique value proposition and market dynamics.
Challenges in Crypto Trading
The primary challenge in crypto trading is its inherent volatility. Factors such as regulatory changes, technological advancements, market sentiment, and global economic shifts can lead to drastic price fluctuations within short time frames.
Table 1: Factors Influencing Crypto Volatility
Factor | Description |
---|---|
Regulatory Changes | Government policies and regulations can impact crypto prices. |
Technological Shifts | Innovations can either boost or hinder a cryptocurrency’s value. |
Market Sentiment | Public perception can drive prices up or down. |
Economic Events | Global events can influence crypto market dynamics. |
The Power of Data-Driven Insights
Data-driven insights provide traders with a structured approach to understand market behaviors. By analyzing vast amounts of data, traders can identify patterns, forecast trends, and make informed decisions. This approach reduces reliance on intuition and enhances the predictability of trading outcomes.
Tools and Techniques for Data Analysis
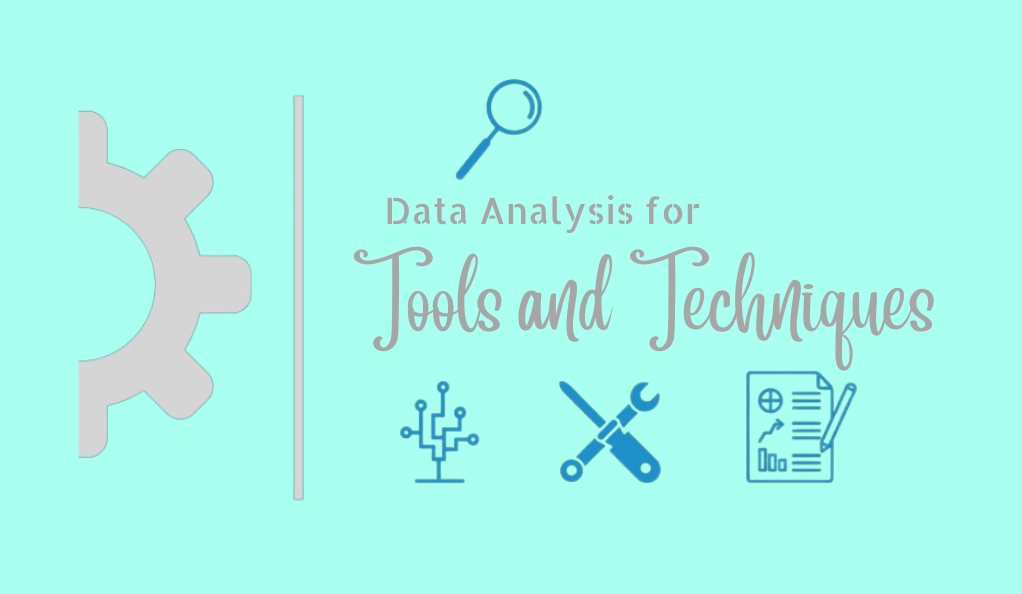
Several tools and techniques are employed to extract insights from crypto data:
- Predictive Analytics: Uses historical data to forecast future price movements.
- Machine Learning: Algorithms that learn from data to predict outcomes.
- Sentiment Analysis: Gauges public sentiment by analyzing online content.
Comparison: Traditional vs. Data-Driven Trading
Table 2: Trading Approaches Compared
Criteria | Traditional Trading | Data-Driven Trading |
---|---|---|
Basis | Intuition and experience | Data analysis and algorithms |
Predictability | Moderate | High |
Tools Used | Fundamental and basic technical analysis | Advanced analytics, machine learning, sentiment analysis |
Risk | Higher due to reliance on intuition | Reduced with data-backed decisions |
Cryptocurrency and Its Inherent Volatility
The Nature of Cryptocurrencies
Cryptocurrencies are digital or virtual currencies that use cryptography for security, making them resistant to counterfeiting. Unlike traditional currencies issued by governments, cryptocurrencies operate on decentralized platforms based on blockchain technology. This decentralization means they are not controlled by any central authority, leading to a unique set of market dynamics.
Factors Driving Price Fluctuations
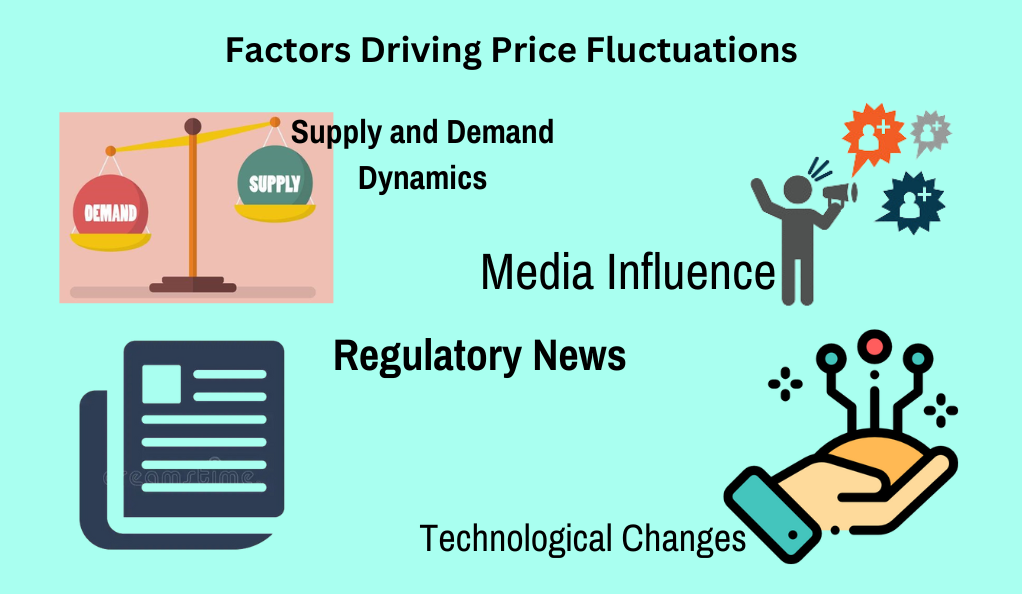
Several factors contribute to the volatility of cryptocurrencies:
- Supply and Demand Dynamics: Limited supply of certain cryptocurrencies, combined with increasing demand, can lead to sharp price increases.
- Regulatory News: Announcements regarding regulations or bans can impact prices significantly.
- Media Influence: News stories or endorsements can lead to increased buying or selling.
- Technological Changes: Innovations or security breaches can influence investor sentiment.
Table 1: Key Factors Influencing Crypto Volatility
Factor | Impact on Price |
---|---|
Supply & Demand | Limited supply and high demand can drive prices up. |
Regulatory News | Positive or negative news can lead to price spikes or drops. |
Media Influence | Media coverage can sway public sentiment. |
Technological Changes | Innovations can boost value, while breaches can decrease it. |
Opportunities in Crypto Trading
The volatility of cryptocurrencies, while posing challenges, also presents opportunities:
- High Returns: The potential for significant price swings allows for substantial profit opportunities.
- Diversification: Cryptocurrencies can be a valuable addition to a diversified investment portfolio.
- Liquidity: High trading volumes mean traders can buy or sell their assets quickly.
Risks and Challenges
However, the volatile nature of cryptocurrencies also introduces risks:
- Potential for Loss: Rapid price declines can lead to significant losses.
- Regulatory Uncertainty: Changing regulations can impact prices and the legality of trading.
- Security Concerns: Cryptocurrencies are susceptible to hacks and security breaches.
Navigating the Volatile Waters
To successfully trade cryptocurrencies, one must stay informed, diversify investments, and employ risk management strategies. Utilizing tools like stop-loss orders and setting clear profit targets can help mitigate potential losses.
The Role of Data Science in Deciphering Cryptocurrency Markets
Understanding Data Science in the Context of Cryptocurrency
Data science is an interdisciplinary field that uses scientific methods, algorithms, and systems to extract insights from structured and unstructured data. In the realm of cryptocurrency, it provides a systematic approach to understanding market behaviors, predicting future trends, and formulating effective trading strategies.
Techniques in Data Science for Cryptocurrency Analysis
Several techniques are employed in data science to analyze cryptocurrency data:
- Statistical Analysis: Examines data to identify patterns, trends, and relationships.
- Data Inference: Draws conclusions from data, often using statistical methods.
- Algorithm Computation: Uses algorithms to process data, make predictions, and automate trading decisions.
Extracting Value from Crypto Data
Data science techniques play a crucial role in extracting value from vast amounts of crypto data:
- Predictive Modeling: Uses historical data to forecast future price movements.
- Sentiment Analysis: Analyzes public sentiment from online content to gauge market mood.
- Anomaly Detection: Identifies unusual patterns that may indicate fraudulent activity or market manipulation.
Table 1: Data Science Techniques and Their Value Extraction
Technique | Value Extraction |
---|---|
Predictive Modeling | Forecasts future trends based on historical data. |
Sentiment Analysis | Gauges market mood to predict price movements. |
Anomaly Detection | Detects unusual patterns indicating potential market risks. |
The Transformative Impact on Trading Strategies
Data science has revolutionized cryptocurrency trading in several ways:
- Informed Decision Making: Traders can make decisions backed by data-driven insights rather than relying solely on intuition.
- Risk Management: Predictive models can help traders set stop-loss orders and manage their portfolios more effectively.
- Automated Trading: Algorithms can automate trading decisions, allowing for high-frequency trading and optimizing returns.
The Road Ahead: Future Implications
As the cryptocurrency market continues to evolve, the role of data science will only become more pronounced. Advanced techniques, such as deep learning and neural networks, will further refine trading strategies. Moreover, as more data becomes available, the insights derived will be even more precise, leading to more effective and profitable trading strategies.
Predictive Analytics: The Future of Crypto Trading
The Essence of Predictive Analytics
Predictive analytics refers to the use of data, statistical algorithms, and machine learning techniques to identify the likelihood of future outcomes based on historical data. In the context of crypto trading, it provides traders with a glimpse into potential future price movements, enabling proactive decision-making.
Forecasting Cryptocurrency Price Movements
The volatile nature of cryptocurrencies makes predicting their price movements a complex endeavor. However, predictive analytics offers a structured approach:
- Historical Data Analysis: Examining past price trends to forecast future movements.
- Market Sentiment Analysis: Gauging public sentiment to predict potential price shifts.
- External Factors: Considering global events, regulatory changes, and technological advancements that might influence prices.
Advanced Algorithms in Predictive Analytics
Modern predictive analytics heavily relies on sophisticated algorithms:
- Machine Learning Algorithms: These learn from data, refining their predictions over time. Examples include regression algorithms, decision trees, and neural networks.
- Time Series Analysis: Specifically designed to analyze time-ordered data, helping to predict future price points based on past patterns.
The Role of Statistical Techniques
Statistical techniques form the backbone of predictive analytics:
- Descriptive Statistics: Provides a summary of the main aspects of the data, such as mean, median, and variance.
- Probability Distributions: Helps in understanding the distribution of data points and the likelihood of different outcomes.
- Hypothesis Testing: Determines if a certain prediction or observation is a result of chance or an actual trend.
Table 1: Key Statistical Techniques in Predictive Analytics
Technique | Application in Crypto Trading |
---|---|
Descriptive Statistics | Summarizing data to understand past trends. |
Probability Distributions | Predicting the likelihood of price movements. |
Hypothesis Testing | Validating the significance of observed trends. |
Implications for Crypto Traders
The integration of predictive analytics in crypto trading has several implications:
- Informed Trading Decisions: Traders can base their decisions on data-driven insights, reducing reliance on intuition.
- Risk Mitigation: Predictive models can help traders set protective measures, such as stop-loss orders.
- Portfolio Optimization: Traders can diversify and adjust their portfolios based on predicted market movements.
Machine Learning: Enhancing Predictive Power
Machine Learning: A Brief Overview
Machine learning, a subset of artificial intelligence, involves algorithms that improve automatically through experience. It uses statistical techniques to give computers the ability to “learn” from data without being explicitly programmed.
The Symbiosis of Machine Learning and Crypto Trading
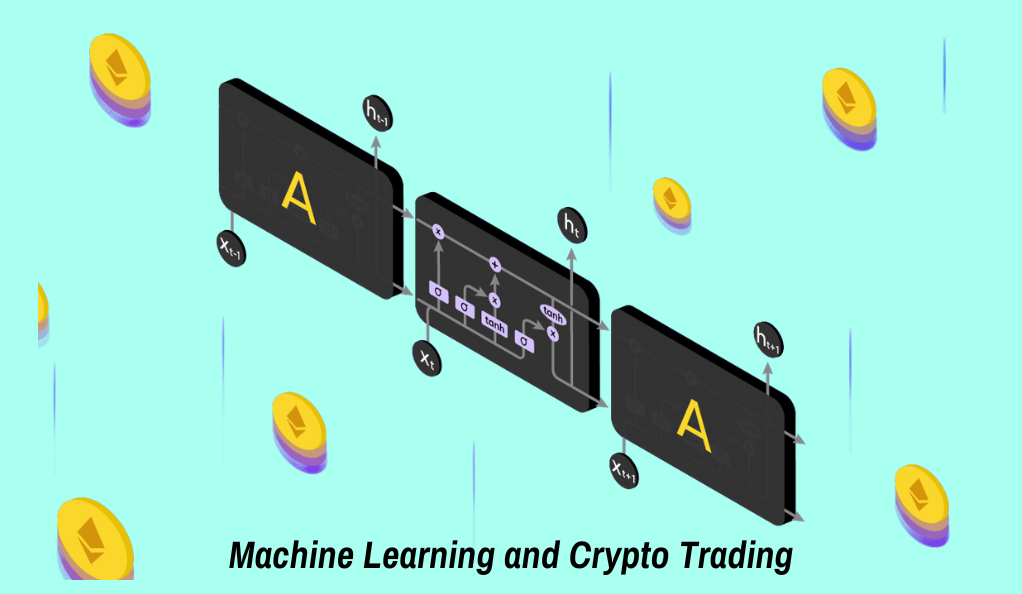
The integration of machine learning in crypto trading offers several advantages:
- Automated Trading: Algorithms can make trading decisions in real-time based on data, allowing for high-frequency trading.
- Pattern Recognition: Machine learning excels at identifying patterns in vast datasets, making it invaluable for spotting trends in price data.
- Risk Management: Predictive models can help traders set protective measures and optimize their portfolios.
Techniques and Algorithms in Play
Several machine learning techniques are employed in crypto trading:
- Supervised Learning: Uses labeled data to predict outcomes. Common algorithms include linear regression and support vector machines.
- Unsupervised Learning: Deals with unlabeled data to find hidden patterns. Clustering and association are typical techniques.
- Reinforcement Learning: An algorithm learns by interacting with an environment and receiving feedback.
Table 1: Machine Learning Techniques in Crypto Trading
Technique | Application in Crypto Trading |
---|---|
Supervised Learning | Predicting price movements based on historical data. |
Unsupervised Learning | Identifying hidden patterns and correlations in price data. |
Reinforcement Learning | Optimizing trading strategies through trial and error. |
Analyzing Historical Price Movements
Machine learning’s ability to analyze past price movements is transformative:
- Feature Extraction: Identifying which factors (e.g., trading volume, market sentiment) have historically influenced prices.
- Time Series Forecasting: Using past price data to predict future price points.
- Anomaly Detection: Spotting unusual price movements that may indicate market manipulation or significant news events.
The Road Ahead: Future Innovations
As technology advances, the role of machine learning in crypto trading is set to expand:
- Deep Learning: Neural networks with multiple layers that can process vast amounts of data for more accurate predictions.
- Natural Language Processing: Analyzing news articles, social media, and other textual data to gauge market sentiment.
- Real-time Analysis: Instantly processing live data feeds to make immediate trading decisions.
Sentiment Analysis: Understanding Market Moods
Sentiment analysis, often referred to as opinion mining, involves processing text to determine the sentiment behind it. It categorizes opinions in text, determining whether the writer’s intention is positive, negative, or neutral.
The Significance in Cryptocurrency Trading
In the volatile world of cryptocurrencies, understanding public sentiment can be a game-changer:
- Price Movement Predictions: Public sentiment can often precede price movements, offering traders a predictive edge.
- Risk Management: Gauging negative sentiment can help traders set protective measures in anticipation of potential market downturns.
- Investment Opportunities: Positive sentiment can highlight potential investment opportunities in emerging cryptocurrencies.
Natural Language Processing (NLP) in Sentiment Analysis
NLP, a branch of artificial intelligence, focuses on the interaction between computers and human language. In sentiment analysis, NLP plays a pivotal role:
- Text Processing: Extracting and processing relevant information from vast amounts of textual data.
- Context Understanding: Determining the context behind words to accurately gauge sentiment.
- Language Variations: Accounting for nuances, slang, and idioms in different languages and regions.
Machine Learning’s Role in Deciphering Sentiments
Machine learning complements NLP in sentiment analysis:
- Training Models: Using labeled datasets to train models that can categorize sentiments in new, unlabeled data.
- Pattern Recognition: Identifying recurring patterns in sentiments related to specific events or news.
- Continuous Learning: Refining models over time as they process more data, improving accuracy.
The Future of Sentiment Analysis in Crypto Trading
As technology evolves, sentiment analysis will see further advancements:
- Real-time Analysis: Instant sentiment gauging from live news feeds, social media, and forums.
- Deep Learning: Using neural networks to understand complex sentiments and their potential market impact.
- Multimodal Sentiment Analysis: Incorporating non-textual data, like audio or video, to provide a holistic sentiment overview.
Case Studies: Real-world Applications of Data Science in Crypto
The Confluence of Data Science and Cryptocurrency
Data science, with its ability to extract meaningful insights from vast datasets, has found a natural ally in the cryptocurrency domain. The decentralized and digital nature of cryptocurrencies generates a wealth of data, ripe for analysis and insight extraction.
Case Study 1: Chainalysis
Overview: Chainalysis is a blockchain analysis company providing data, software, and expertise on cryptocurrencies.
Application of Data Science:
- KYT (Know Your Transaction): Chainalysis uses data science to monitor large volumes of cryptocurrency activity and identify high-risk transactions.
- Market Intel: Leveraging data analytics to provide insights into cryptocurrency activities, helping businesses and authorities understand market trends.
Case Study 2: Elliptic
Overview: Elliptic specializes in identifying and preventing illicit activity in cryptocurrencies.
Application of Data Science:
- Transaction Monitoring: Uses machine learning to identify patterns associated with illicit activities like money laundering.
- Risk Assessment: Analyzes transactions to gauge the risk associated with different cryptocurrency wallets and addresses.
Case Study 3: Nomics
Overview: Nomics is a crypto market cap & pricing data provider.
Application of Data Science:
- Transparent Volume: Uses data science to provide a more transparent volume metric for cryptocurrencies, filtering out “fake” or “wash” trading.
- Historical Data Analysis: Offers comprehensive historical data, allowing traders and researchers to analyze past market trends.
Beyond Financial Analytics: Innovative Applications
Data science’s application in the crypto sector isn’t limited to financial analytics:
- Smart Contract Analysis: Using data analytics to assess the performance and security of smart contracts.
- Tokenomics Research: Analyzing data to understand the distribution, ownership, and usage of different cryptocurrency tokens.
- Community Sentiment Analysis: Gauging the sentiment of cryptocurrency communities to predict potential market movements.
The Road Ahead: Future Prospects
As the crypto sector continues to mature, the applications of data science will expand:
- Decentralized Finance (DeFi) Analysis: With the rise of DeFi platforms, data analytics will play a crucial role in understanding and optimizing these decentralized financial systems.
- Inter-chain Analysis: As interoperability between different blockchains becomes more prevalent, data science will be pivotal in analyzing cross-chain data.
Conclusion
The intersection of data science and the cryptocurrency realm has ushered in a transformative era, marked by enhanced insights and innovative applications. As showcased through real-world case studies, businesses are not only leveraging data science to optimize trading and financial analytics but are also pioneering its use in areas like smart contract analysis, tokenomics research, and community sentiment gauging. The symbiotic relationship between these two domains promises a future where data-driven decisions will shape the crypto landscape, ensuring its growth, security, and sustainability. As we navigate this evolving digital financial frontier, the fusion of data science and cryptocurrency stands as a beacon, guiding the way towards a more informed and integrated future.
Bitcoin-up is dedicated to providing fair and trustworthy information on topics such as cryptocurrency, finance, trading, and stocks. It's important to note that we do not have the capacity to provide financial advice, and we strongly encourage users to engage in their own thorough research.
Read More